The financial industry is undergoing a digital transformation, and Artificial Intelligence (AI) is at the center of this revolution. From fraud detection to algorithmic trading and personalized financial advice, AI and machine learning (ML) are driving innovation, efficiency, and accuracy.
AI in finance enables companies to process vast amounts of data, identify patterns, and make smarter decisions in real-time. As financial institutions adapt to an increasingly digital world, machine learning applications are enhancing risk management, automating tasks, and improving customer experiences.
In this blog, we’ll explore how machine learning is transforming the finance industry, its key applications, and the benefits it offers for businesses and consumers alike.
Why AI and Machine Learning Are Crucial in Finance
The finance sector deals with immense amounts of data, from transactions to credit histories, market trends, and customer behavior. Traditional methods for analyzing such data are often slow, inefficient, and prone to errors.
AI and machine learning provide solutions to these challenges through:
- Speed: Processing millions of transactions or market signals in seconds.
- Accuracy: Reducing errors by learning from historical data and patterns.
- Scalability: Managing large datasets across financial institutions effortlessly.
- Automation: Streamlining repetitive processes to save time and costs.
By leveraging AI, financial organizations are making more informed, data-driven decisions, improving security, and delivering seamless customer experiences.
Key Applications of Machine Learning in Finance
1. Fraud Detection and Prevention
Financial fraud is a growing concern, with cybercriminals using increasingly sophisticated techniques. Machine learning algorithms analyze historical data and real-time transaction patterns to identify unusual activities.
How It Works:
- AI models detect anomalies such as large withdrawals, irregular purchases, or duplicate transactions.
- Machine learning improves over time, learning new fraud patterns and minimizing false positives.
Example: Banks use AI to block unauthorized transactions immediately, ensuring customers’ funds remain secure.
Benefits:
- Faster fraud detection.
- Improved security for businesses and customers.
2. Credit Scoring and Risk Assessment
Traditional credit scoring relies heavily on limited historical financial data. Machine learning enhances this process by considering multiple data points, including alternative credit signals.
How It Works:
- ML models analyze credit histories, spending behaviors, social signals, and even employment data.
- Real-time scoring ensures accurate risk assessment, even for first-time borrowers.
Example: Fintech companies like Upstart use AI-driven credit scoring to approve loans for individuals overlooked by traditional lenders.
Benefits:
- Accurate risk profiling.
- Increased financial inclusion for underserved populations.
3. Algorithmic Trading
AI and machine learning are transforming stock markets with algorithmic trading, which uses predictive analytics to execute trades faster and more accurately than humans.
How It Works:
- ML algorithms analyze historical market data, news trends, and real-time signals.
- AI identifies opportunities for buying or selling stocks to maximize returns.
Example: Hedge funds and investment firms use AI-driven bots for high-frequency trading (HFT) to make split-second trading decisions.
Benefits:
- Faster and smarter trading strategies.
- Improved investment returns.
4. Personalized Financial Services
AI enables financial institutions to deliver personalized experiences by understanding customer preferences and behaviors.
How It Works:
- Machine learning models analyze user data to offer tailored financial advice.
- AI-powered virtual assistants help customers with budgeting, savings goals, and investments.
Example: Robo-advisors like Betterment and Wealthfront use AI to provide personalized investment portfolios at lower costs.
Benefits:
- Enhanced customer satisfaction.
- Access to financial planning tools for all income levels.
5. Fraud Risk Prediction and Anti-Money Laundering (AML)
Machine learning improves anti-money laundering systems by identifying suspicious patterns in large volumes of transactions.
How It Works:
- AI scans transaction records to spot money laundering schemes and other financial crimes.
- ML algorithms continuously learn new techniques used by fraudsters.
Example: Financial institutions use AI-powered AML tools to comply with regulations and prevent illicit activities.
Benefits:
- Enhanced regulatory compliance.
- Reduced manual workload for compliance teams.
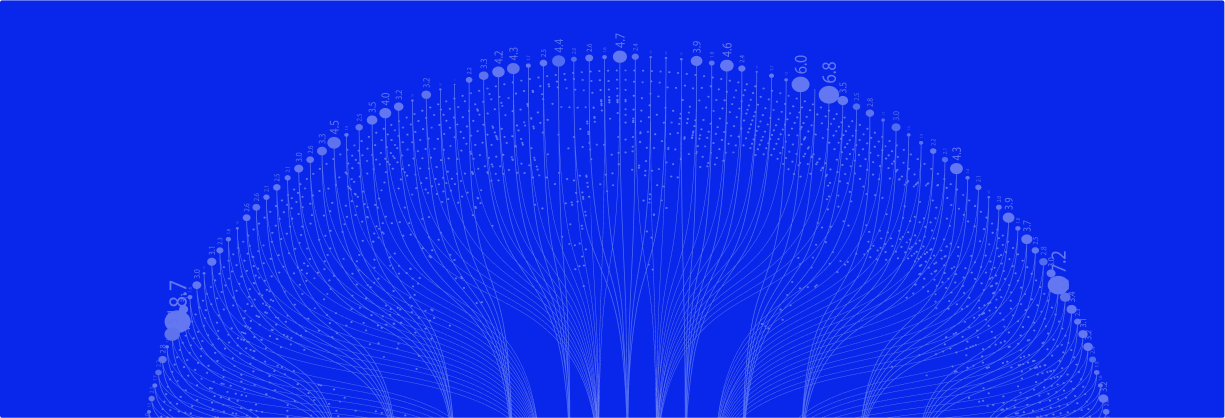
Benefits of AI in the Finance Industry
1. Improved Decision-Making
AI provides real-time insights by analyzing massive datasets, enabling organizations to make smarter decisions faster.
2. Cost Efficiency
Automation of repetitive processes reduces operational costs and increases efficiency.
3. Enhanced Security
AI improves fraud detection and enhances cybersecurity, protecting financial institutions and their customers.
4. Customer-Centric Solutions
AI-driven personalization delivers better experiences, improving customer loyalty and trust.
5. Scalability
AI systems can process and analyze vast amounts of data, ensuring seamless operations for financial institutions.
Challenges of Implementing AI in Finance
While the benefits of AI are clear, financial organizations face challenges, including:
- Data Privacy Concerns: Financial data is sensitive, requiring strict security and compliance measures.
- Regulatory Barriers: AI applications must comply with evolving legal frameworks across regions.
- Bias in Algorithms: Poorly trained AI models can produce biased decisions, affecting customers unfairly.
- Integration Costs: Implementing AI requires significant investment in infrastructure and skilled personnel.
To address these challenges, businesses need to adopt ethical AI practices, invest in secure systems, and ensure transparency in decision-making processes.
The Future of AI in Finance
The adoption of AI in finance will continue to grow, driven by innovation and increasing customer demands. Key trends include:
- Explainable AI (XAI): Financial institutions will adopt transparent AI systems that explain decisions to customers and regulators.
- AI-powered Predictive Analytics: Predicting market trends, customer behaviors, and economic shifts with greater accuracy.
- Blockchain Integration: Combining AI with blockchain to enhance security and transparency in transactions.
- AI-driven Financial Inclusion: Using AI to provide financial services to underserved populations worldwide.
Conclusion
AI and machine learning are revolutionizing the financial industry, offering innovative solutions to challenges like fraud detection, risk assessment, and personalized customer services. By enabling smarter decisions, automating processes, and enhancing security, AI is not only improving financial services but also shaping a more inclusive and efficient future for the industry.
While challenges remain, organizations that invest in AI-driven solutions will gain a competitive edge, driving growth and innovation in a dynamic market.