Artificial Intelligence (AI) is advancing at an unprecedented pace, yet its development is not without challenges. From high computational demands and limited data availability to addressing ethical concerns and reducing bias, current AI training techniques face significant hurdles. However, a wave of innovative methodologies is emerging to address these obstacles. This blog explores the latest training techniques revolutionizing AI and paving the way for more efficient, ethical, and scalable systems.
Challenges in Current AI Training Techniques
- Data Dependency and Scarcity:
- AI models often require massive datasets, which are not always readily available, especially in niche domains.
- Data collection and labeling are time-intensive and costly.
- Computational Costs:
- Training advanced models like GPT-4 demands enormous computational power, leading to high energy consumption and environmental concerns.
- Model Bias and Fairness Issues:
- Training datasets can inadvertently encode societal biases, resulting in biased AI outputs.
- Ensuring fairness and inclusivity in AI decision-making remains a complex challenge.
- Overfitting and Generalization:
- Models trained on specific datasets often struggle to generalize to new, unseen data.
- Lack of Explainability:
- Many AI models, especially deep learning systems, operate as “black boxes,” making it difficult to understand their decision-making processes.
Emerging AI Training Techniques
- Few-Shot and Zero-Shot Learning:
- Minimize data dependency by training models to learn from a small number of examples or none at all.
- Examples: GPT-4’s ability to perform tasks without specific task-related fine-tuning.
- Federated Learning:
- Decentralized training allows models to learn from data stored across multiple devices without transferring it to a central server.
- Improves data privacy and reduces security risks.
- Self-Supervised Learning:
- Models generate their own labels from raw data, reducing reliance on manually labeled datasets.
- Examples include BERT and other transformer-based architectures.
- Energy-Efficient Training:
- Techniques like sparse training and quantization optimize models for lower energy consumption without compromising performance.
- Advances in hardware, such as neuromorphic chips, also play a crucial role.
- Adversarial Training:
- Enhance model robustness by exposing AI to adversarial examples during training.
- Reduces vulnerability to adversarial attacks and improves model reliability.
- Ethical AI Frameworks:
- Incorporate fairness metrics during training to mitigate bias.
- Use synthetic data generation to create more balanced datasets.
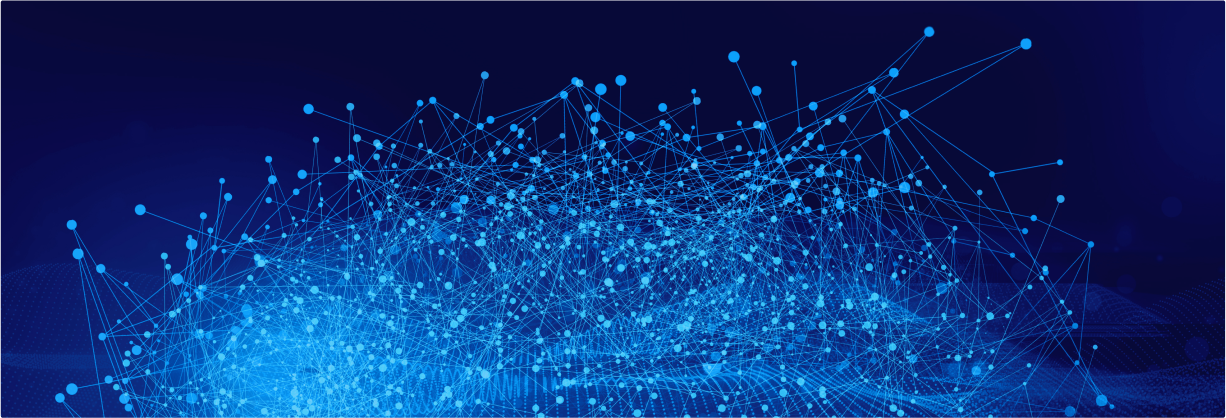
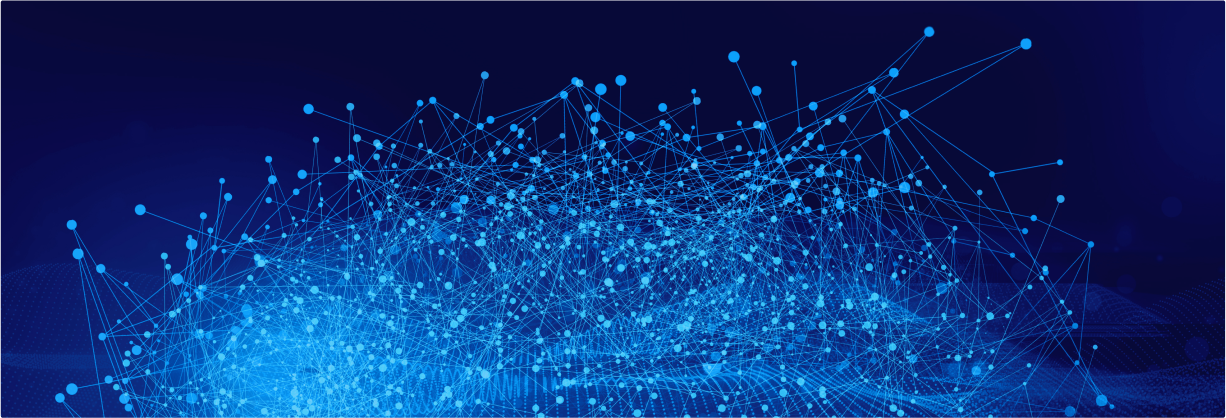
Benefits of These Innovations
- Efficiency Gains:
- Reduced computational costs and training times allow broader accessibility to AI technologies.
- Scalability:
- Decentralized and energy-efficient methods enable the deployment of AI in resource-constrained environments.
- Improved Ethics and Trust:
- Bias mitigation and transparency techniques foster public trust in AI systems.
- Wider Applicability:
- Few-shot and zero-shot techniques empower AI to excel in domains with limited data availability.
- Resilience and Robustness:
- Adversarial training ensures models perform reliably in dynamic, real-world conditions.
The Road Ahead
As AI becomes more integral to industries and everyday life, the need for smarter, faster, and fairer training techniques will grow. Future developments may include:
- Neuro-Symbolic AI:
- Combining symbolic reasoning with neural networks for more interpretable and efficient AI systems.
- Lifelong Learning Models:
- AI systems capable of continuous learning from new data without forgetting prior knowledge.
- Synthetic Data Innovation:
- Leveraging generative AI to create realistic datasets for training, bridging data gaps across domains.
- Explainable AI (XAI):
- Developing models that can provide human-understandable explanations for their decisions.
Conclusion:
AI is entering a transformative phase where training techniques are evolving to address longstanding challenges. From improving efficiency and scalability to enhancing fairness and robustness, these innovations promise to make AI more accessible, reliable, and ethical. By embracing these cutting-edge methodologies, the AI community is shaping a future where intelligent systems can better meet the needs of businesses, researchers, and society at large.